Consider the following:
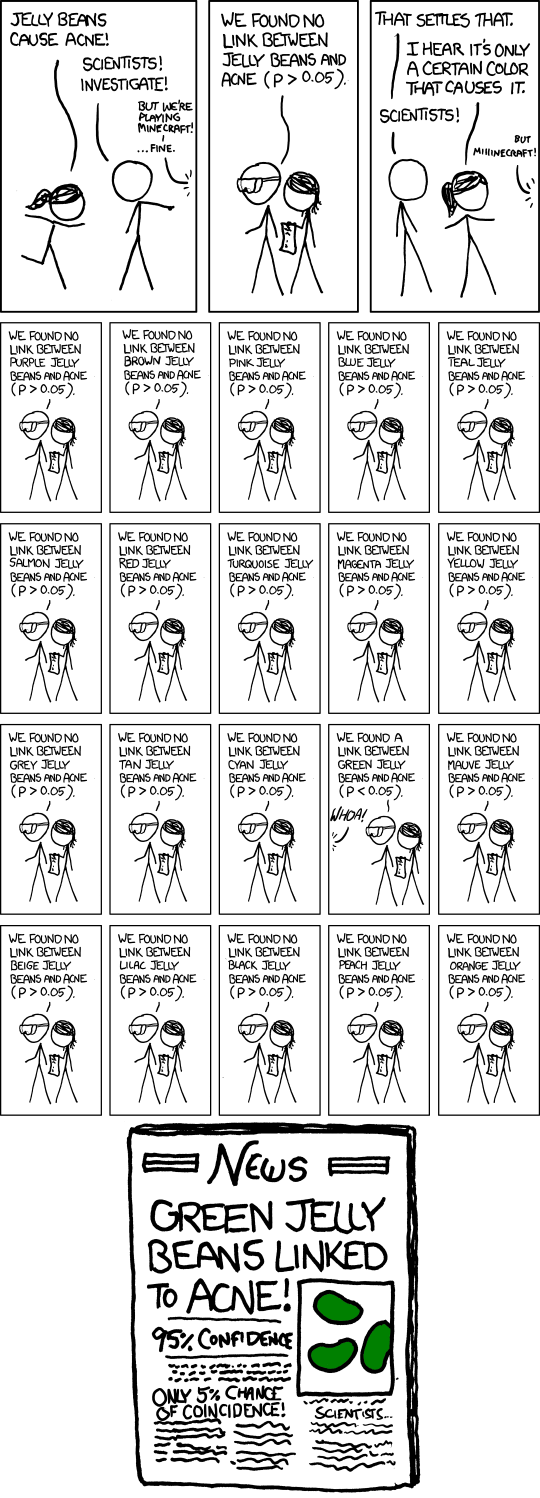
Obviously this is bad science and even worse scientific reporting, but what can be done to combat it? More generally, what should be the scholarly response to the growing sense, among scientific researchers and the lay public alike, that scientific publications are not trustworthy — that is, that the report of a statistically significant finding in a reputable scientific journal does not in general warrant drawing any meaningful conclusions?
A new paper in the journal Nature Human Behavior proposes a simple but radical solution: the default P-value threshold for statistical significance should be changed from 0.05 to 0.005 for claims of new discoveries.
The paper has dozens of co-authors, many of them quite distinguished. Given both the importance of the topic and the attention that the paper has already generated, it seemed worth organizing a discussion of the paper here at Brains. Below you’ll find just that: following a précis by the paper’s lead authors there is a series of commentaries, by Felipe De Brigard (Duke), Kenny Easwaran (Texas A&M), Andrew Gelman (Columbia) and Blake McShane (Northwestern), Kiley Hamlin (UBC), Edouard Machery (Pittsburgh), Deborah Mayo (Virginia Tech), the neuroscientist who blogs under the pseudonym Neuroskeptic, Michael Strevens (NYU), and Kevin Zollman (Carnegie Mellon University). Each can be viewed by clicking on the author’s (or authors’) names.
This post will be open for discussion for a couple of weeks. Many thanks to all those involved!
***
[expand title=”Précis by Dan Benjamin, Jim Berger, Magnus Johannesson, Valen Johnson, Brian Nosek, and EJ Wagenmakers:”]
Researchers representing a wide range of disciplines and statistical perspectives—72 of us in total—have published a paper in Nature Human Behavior describing a place of common ground. We argue that statistical significance should be redefined.
For claims of discoveries of novel effects, the paper advocates a change in the P-value threshold for a “statistically significant” result from 0.05 to 0.005. Results currently called “significant” that do not meet the new threshold would be called suggestive and treated as ambiguous as to whether there is an effect. The idea of changing the statistical significance threshold to 0.005 has been proposed before, but the fact that this paper is authored by statisticians and scientists from a range of disciplines—including psychology, economics, sociology, anthropology, medicine, epidemiology, ecology, and philosophy—indicates that the proposal now has broad support.
The paper highlights a fact that statisticians have known for a long time but which is not widely recognized in many scientific communities: evidence that is statistically significant at P = 0.05 actually constitutes fairly weak evidence. For example, for an experiment testing whether there is some effect of a treatment, the paper reports calculations of how different P-values translate into the odds that there is truly an effect vs. not. A P-value of 0.05 corresponds to odds that there is truly an effect that range, depending on assumptions, from 2.5:1 to 3.4:1. These odds are low, especially for surprising findings that are unlikely to be true positives in the first place. In contrast, a P-value of 0.005 corresponds to odds that there is truly an effect that range from 14:1 to 26:1, which is far more convincing.
An important impetus for the proposal is the growing concern that there is a “reproducibility crisis” in many scientific fields that is due to a high rate of false positives among the originally reported discoveries. Many problems (such as multiple hypothesis testing and low power) have contributed to this high rate of false positives, and we emphasize that it is important to address all of these problems. We argue, however, that tightening the standards for statistical significance is a simple step that would help. Indeed, the theoretical relationship between the P-value and the strength of the evidence is empirically supported: the lower the P-value of the reported effect in the original study, the more likely the effect was to be replicated in both the Reproducibility Project Psychology and the Experimental Economics Replication Project.
Lowering the significance threshold is a strategy that has previously been used successfully to improve reproducibility in several scientific communities. The genetics research community moved to a “genome-wide significance threshold” of 5×10-8 over a decade ago, and the adoption of this standard helped to transform the field from one with a notoriously high false positive rate to one with a strong track record of robust findings. In high-energy physics, the tradition has long been to define significance for new discoveries by a “5-sigma” rule (roughly a P-value threshold of 3×10-7). The fact that other research communities have maintained a norm of significance thresholds more stringent than 0.05 suggests that transitioning to a more stringent threshold can be done.
Changing the significance threshold from 0.05 to 0.005 carries a cost, however: Apart from the semantic change in how published findings are described, the proposal also entails that studies should be powered based on the new 0.005 threshold. Compared to using the old 0.05 threshold, maintaining the same level of statistical power requires increasing sample sizes by about 70%. Such an increase in sample sizes means that fewer studies can be conducted using current experimental designs and budgets. But the paper argues that under realistic assumptions, the benefit would be large: false positive rates would typically fall by factors greater than two. Hence, considerable resources would be saved by not performing future studies based on false premises. Increasing sample sizes is also desirable because studies with small sample sizes tend to yield inflated effect size estimates, and publication and other biases may be more likely in an environment of small studies.
In research communities where attaining larger sample sizes is simply infeasible (e.g., anthropological studies of a small-scale society), there is a related “cost”: most findings may no longer be statistically significant under the new definition. Our view is that this is not really a cost at all: calling findings with P-values in between 0.05 and 0.005 “suggestive” is actually a more accurate description of the strength of the evidence.
Indeed, the paper emphasizes that the proposal is about standards of evidence, not standards for policy action nor standards for publication. Results that do not reach the threshold for statistical significance (whatever it is) can still be important and merit publication in leading journals if they address important research questions with rigorous methods. Evidence that does not reach the new significance threshold should be treated as suggestive, and where possible further evidence should be accumulated. Failing to reject the null hypothesis (still!) does not mean accepting the null hypothesis.
The paper anticipates and responds to several potential objections to the proposal. A large class of objections is that the proposal does not address the root problems, which include multiple hypothesis testing and insufficient attention to effect sizes—and in fact might reinforce some of the problems, such as the over-reliance on null hypothesis significance threshold and bright-line thresholds. We essentially agree with these concerns. The paper stresses that reducing the P-value threshold complements—but does not substitute for—solutions to other problems, such as good study design, ex ante power calculations, pre-registration of planned analyses, replications, and transparent reporting of procedures and all statistical analyses conducted.
Many of the authors agree that there are better approaches to statistical analyses than null hypothesis significance testing and will continue to advocate for alternatives. The proposal is aimed at research communities that continue to rely on null hypothesis significance testing at a 0.05 threshold; for those communities, reducing the P-value threshold for claims of new discoveries to 0.005 is an actionable step that will immediately improve reproducibility. Far from reinforcing the over-reliance on statistical significance, we hope that the change in the threshold—and the increased use of describing results with P-values between 0.05 and 0.005 as “suggestive”—will raise awareness of the limitations of relying so heavily on a P-value threshold and will thereby facilitate a longer-term transition to better approaches.
The proposed switch to a more demanding P-value threshold involves both a coordination problem (what threshold to use?) and a free-riding problem (why should I impose a more stringent threshold on myself unless others do?). The aim of the proposal is to help coordinate on 0.005 and to discourage free-riding on the old threshold. Ultimately, we believe that the new significance threshold will help researchers and readers to understand and communicate evidence more accurately.
This précis was originally published at https://cos.io/blog/we-should-redefine-statistical-significance/. It is reprinted with permission.
[/expand]
[expand title=”Commentary by Felipe De Brigard:”]
Although it was published just a month ago, Benjamin et al’s (2017) proposal has already been so widely discussed that I find it difficult to say something new about the paper. Instead, in this comment, I want to (1) re-emphasize some points that have been made in response, and (2) say something general about the discussion on statistical significance and replicability.
But, first, a comment about the aim of Benjamin’s paper. What’s the point of arguing that the threshold of statistical significance should really be p < .005 rather than p < .05? After all, as McShane et al (2017) remind us, the p < .05 threshold is arbitrary and lexicographic. It harks back to Neyman and Pearson’s (1933) recommendation, which in turn was based upon Fisher’s (1926) p <.05 cut-off; but even Neyman and Pearson (1928)—as Laken’s et al (2018) reminds us—as well as Fisher (1956) himself, were against using p < .05 as a fixed criterion, let alone a publication cut-off, for all disciplines and independent of experimental conditions.
Benjamin and collaborators are well aware of this. In fact, they clearly state that they don’t mean to suggest that journals should now reject papers whose results don’t reach the new statistical significance level. They do suggest, though, that results at p < .05 level should be called “suggestive” rather than “significant”. Gradability in the use of statistical significance is old news. Considerations about the relationship between p-values and the strength of evidence led some disciplines, such as certain branches of biology, to take p < .05 as “significant”, p < .01 a “very significant” and p < .001 as “highly significant” (Quinn & Keough, 2002). We even adopted pictorial conventions to reflect these “degrees of significance” (e.g., *, **, and ***, respectively). So, if the notion of statistical significance is already this fluid, what would be the purpose of redefining it to mean p < .005?
My sense is that they want to make two points—one more controversial than the other. The less controversial point is that the p < .05 seem to have taken a life of its own in certain sciences—particularly social sciences—leading people to take any H1 surviving a p < .05 threshold as true; or, worse, any H0 that gets rejected at p < .05 as false, no matter how much p-hacking or data-fitting one had to do to get to it. It is as if reaching significance would anoint a result with a patina of truth. It does not, and Benjamin and colleagues make a good case to remind us why this is not the case. Sure, their newly proposed p < .005 threshold is merely “lexicographic”, as McShane et al argue, but words matter a lot, and it is important that we know when to use them and what they mean.
The second point is, however, more controversial. They suggest that redefining statistical significance to p < .005 would “immediately improve the reproducibility of scientific research in many fields”. Most of the responses to Benjamin et al’s paper have been focused on this, more contentious, part of their proposal, and I agree with some of them. Perhaps my biggest worry—which was mentioned by Lakens et al (2017) and Mayo (2017)—is that their proposal relies on the very difficult construct of prior odds, which in turn is defined upon the probability that H0 is true (φ). The problem is that both φ and, by extension, the prior odds as defined by the equation in Fig. 2, are highly dependent on factors that shouldn’t affect the degree to which a result is deemed statistically significant—at least ideally. For instance, if the prior odds are calculated by surveying a sample of papers from a particular set of journals within a discipline, the odds that there may be publication bias toward certain views are, I’d say, pretty high. I don’t think it comes as a surprise that many editors and reviewers are biased toward publishing certain kinds of papers that support certain kinds of views; even without having a “dog in that fight” they may be biased against accepting papers that do not support “well stablished findings”. As a result, the distribution from which φ is supposed to be derived may be skewed, and it could end up favoring—that is, giving high prior odds to—a H0 that may likely be false (Mayo, 2018)[1].
Despite these concerns, Benjamin et al’s paper is important, thought provoking, and I learned a lot from it. And so are the responses it ensued. So, what should we do: should we redefine (Benjamin et al., 2017), remove (Amrhein and Greenlad, 2017), justify (Lakens et al., 2017) or abandon (McShane et al., 2017) statistical significance? My sense is that the preferred strategy –or strategies, because they are not mutually exclusive—is going to depend on the specific project. What is clear, though, is that all these proposals share a general motivation: the betterment of scientific practice. And my hunch is that they all come from a similar worry: we, scientists, are overreaching. We are constantly making generalizations that go beyond what the data really supports, not only because we report our “statistically significant” results as if they have uncovered something astonishingly true about our sample, but also because we often unjustifiably generalize from our sample to a larger population.
I think this is something all these papers agree on: we need to be humbler in our claims about what the evidence shows, we should be careful when interpreting novel findings at p < .05 with low priors, or underpowered studies, or experiments with too many researcher degrees of freedom (Simmons et al, 2011). Likewise, we should also educate ourselves more in the particular choices of models we make, so we can better justify our alpha levels (Lakens et al, 2017), specially in this time and age in which scientific data are becoming more and more complex, which both affords better signal but also more noise. And we should also be happier embracing the uncertainty of scientific practice and its results (McShane et al, 2017). These are all useful practical suggestions, and it would be great if they become habits.
To these, I would just add one last recommendation: we need to be much humbler about the reach of our conclusions when there is uncertainty as to what is the population our sample is representative of. For us working in psychology and cognitive science, it is not uncommon to receive reports from reviewers doubting the validity of a result in which one fails to find a certain effect, on account that the sample employed may have come from a population different from the original finding. I know of a replication attempt that got rejected because the sample the researchers employed was different from that used in the original study. What was strange is that the original paper was making claims about people, and all of the participants employed in the replication attempt were, well, people, so it is not unreasonable to believe that they were drawing from the same population. Of course, I am being facetious. This is probably not what the reviewer had in mind. What s/he probably had in mind is that the original experiment was conducted with, say, MBA students, while the replication attempt was done with MTurkers. Fair enough. Yet, I couldn’t help but wonder: if the sample the first authors employed (i.e., MBA students) wasn’t really representative of the population they claimed their results were about (i.e., people), and there was reason to believe that the effect wouldn’t hold for some other sample of the same population (i.e., MTurkers), why was it ok for them to generalize their “finding” in the first place?
We used not to be like this. The other day I was reading an old paper in the psychology of nostalgia. The author, Dr. Rose from Smith College, writes that 50% of the 66 undergraduate students she interviewed reported feelings of homesickness (Rose, 1947). Nevertheless, she warns her readers, from the very beginning, that “this proportion cannot be taken as indicative of the incidence of homesickness among female college freshmen” (p. 185), and she goes on to explain the particularities of her sample, and the degree to which certain characteristics are or are not generalizable to other female college freshmen. At no point was there a claim about all undergraduates—let alone all people. I think that unjustifiable generalizations from sample to populations are partly responsible for the current crisis of replicability, and I would very much like to go back to our humbler origins, when psychologists payed more attention to representativeness, and less to marketing their results as reaching beyond what they should. So, on top of all of these great proposals about statistical significance I want to suggest one more lexicographic recommendation: let’s stop reporting our results as “findings”. We often don’t know if they are. Not all effects, whether at p < .05 or < .005, or even < .0001, are sufficient to prove that something is there. And, I reckon, for something to be there is a necessary condition for it to be found.
References
Amrhein, V. and Greenlad, A. (2017). Remove, rather than redefine, statistical significance. Nature Human Behavior.
Benjamin, D. et al. (2017). Redefine statistical significance. Nature Human Behavior.
Fisher, R.A. (1926). The arrangement of field experiments. Journal of the Ministry of Agriculture in Great Britain, 33, 503-513.
Fisher, R.A. (1956) Statistical methods and scientific inference. Hafner: NY.
Laken, D. et al. (2017) Justify your alpha: A response to “Redefine statistical significance” https://psyarxiv.com/9s3y6
Mayo, D. (2018). Statistical inference as severe testing: How to get beyond the statistics wars. Cambridge University Press.
McShane, B.B. et al. (2017). Abandon statistical significance. https://www.stat.columbia.edu/ ~gelman/research/unpublished/abandon.pdf
Neyman, J. and Pearson, E.S. (1928). On the use and interpretation of certain test criteria for purposes of statistical inference: Part I. Biometrika, 175-240.
Neyman, J. and Pearson, E.S. (1933). On the problem of the most efficient tests of statistical hypotheses. Philosophical Transactions of the Royal Society of London (A), 231, 289-337.
Quinn, G.P. and Keough, M.J. (2002). Experimental design and data analysis for biologists. Cambridge University Press.
Rose, A.A. (1947). A study of homesickness in college freshmen. The Journal of Social Psychology, 26: 185-202.
Simmons, J.P.,, Nelson, L.D., and Simonsoh, U. (2011). False-positive psychology: Undisclosed flexibility in data collection and analysis allows presenting anything as significant. Psychological Science, 22(11): 1359-1366.
[1] I am, following Laken et al’s, referencing Mayo’s forthcoming book, although I think I read this first on her twitter account.
[/expand]
[expand title=”Commentary by Kenny Easwaran:”]
This paper on redefining statistical significance has over 70 authors signed on to it, from many different academic fields. It thus represents more of a compromise among many different perspectives, than the primary view of many (any?) of the authors on the difficulties facing statistical methodology in practice today. As one of the many middle authors, I read a few drafts and gave a few comments, but my main contribution was a signature.
The central idea of the paper is a call to change the social convention for how to carry out a significance test in mainstream scientific literature. Like many of my co-authors, I actually think that most significance testing in the scientific literature is misused. However, it is useful for there to be conventional formats for scientific studies, and the significance test has become such a convention. A change in the conventional threshold used to indicate significance would avoid some problems for the scientific community that have arisen as a result of the misuse of significance tests. And moreover, a controversy around a call to change this conventional practice may help the scientific community come together around other conventions that may better serve the community. I hope that this paper is having the relevant effect.
The idea of a significance test is that it gives an experimental design for rejecting a null hypothesis, where the pre-arranged structure of the experiment is such that the null hypothesis is likely to be rejected if it is false (with probability given by the “power” of the experiment) and unlikely to be rejected if it is true (with probability given by the chosen p-value required for significance). This structure is ideally suited for making a sequence of final decisions about similar cases, like a sequence of patients to treat or not. One can design the experiment so that the power and pre-selected p-value used line up with the ratio of the harms caused by applying the treatment in a case of the wrong sort, to the harms caused by failing to apply the treatment in a case of the right sort, and the costs of performing an experiment where both types of errors are unlikely.
However, in much scientific practice, individual significance tests are now used as part of a longer series of investigations, which might include further significance tests of the same null hypothesis (against the same or different alternatives), as well as investigations of other forms. In this context, the power and the p-value no longer have the standard meaning, but are instead used as measures of the evidential force of individual studies. This is in some ways analogous to employers treating college degrees as measures of suitability for employment rather than as indicators of a related, but distinct, set of skills and curricular achievements.
As one coauthor, John Ioannidis, has famously observed in many other publications [1], in some fields, the combination of the misuse of significance tests and the high base rate for false hypotheses has led to the acceptance of many false claims. A major section of our paper shows how this problem would decrease under the stricter threshold for significance, even with no further change to the use of significance tests. We further claim that the benefits of the decreased false hypotheses would outweigh the extra costs involved in performing large enough studies to achieve the stricter threshold while maintaining high power, though I suspect much debate among practitioners will focus on whether or not this is really true.
I and some other coauthors believe that many of the purposes currently served by significance tests would be better served by certain Bayesian statistical methods. Of course, there are still philosophical controversies about whether it is possible to use these methods in the objective way we expect science to work. My philosophical work on Bayesianism starts from the presumption that there is an ineliminable subjective component, so there are questions about what sorts of results can be reported in an objective (or at least, intersubjective) way, enabling each researcher to use them appropriately.
As someone who has not directly engaged in the sorts of scientific practice that rely on statistical methods, I am not in the best position to say what is the best conventional form of study to replace significance tests in many of their uses. But given the statistical crises that have faced certain medical and social scientific fields in recent years, I hope that the proposal of this paper can help lead to some improvements.
[1] https://journals.plos.org/
[/expand]
[expand title=”Commentary by Andrew Gelman and Blake McShane:”]
There are a lot of ways to gain replicable quantitative knowledge about the world. In the design stage, we try to gather data that are representative of the population of interest, collected under realistic conditions, with measurements that are accurate and relevant to underlying questions of interest. Over the past century, statisticians and applied researchers have refined methods such as probability sampling, randomized experimentation, and reliable and valid measurement in order to allow them to gather data that ever more directly speaks to the scientific hypotheses and predictions of interest. And each of these protocols is associated with statistical methods to assess systematic and random error, which is necessary given that we are inevitably interested in making inferences that generalize beyond the data at hand. In practice, it is necessary to go even further and introduce assumptions to deal with issues such as missing data, nonresponse, selection based on unmeasured factors, and plain old interpolation and extrapolation.
All the above is basic to statistics, and the usual textbook story–at least until recently–was that it all was working fine. Researchers design experiments, gather data, add some assumptions, and produce inferences and probabilistic predictions which are approximately calibrated. And the scientific community verifies this through out-of-sample tests–that is, replications.
On the ground, though, something else has been happening. Yes, researchers conduct their studies, but often with little regard to reliability and validity of measurement. Why? Because the necessary step on the way to publication and furthering of a line of research was not prediction, not replicable inference, but rather statistical significance–p-values below 0.05–a threshold that was supposed to protect science from false alarms but instead, for reasons of “researcher degrees of freedom” (Simmons, Nelson, and Simonsohn, 2011) or the “garden of forking paths” (Gelman and Loken, 2014) was stunningly easy to attain even from pure noise.
In the past few years, it has been increasingly clear that there are areas of science, including certain subfields of social psychology and medicine, where false alarms (which can be defined as cases in which a strong claim is made that is not–and often cannot be–supported by the data at hand) have been sounding so loudly and frequently that little else can be heard. There are two pieces of evidence to support the claim that these areas of science are broken. First, the use of significance testing as a threshold screening rule in domains with noisy data and abundant researcher degrees of freedom yields claims with exaggerated effect sizes (type M or magnitude errors) that are frequently in the wrong direction (type S or sign errors), as discussed, for example, by Button et al. (2013) and Gelman and Carlin (2014). Second, empirical efforts to replicate published findings have frequently failed (Open Science Collaboration, 2015), with many prominent examples; see, for example, Engber (2016) and Bartels (2017). This combined onslaught from statistical theory and empirical efforts has led to the replication crisis being recognized from nearly all corners of the biomedical and social sciences.
What should be done? In a widely discussed recent paper, Benjamin et al. (2017) recommend replacing the conventional p<0.05 threshold by the more stringent p<0.005 for “claims of new discoveries.” However, because merely changing the p-value threshold cannot alleviate the theoretical issues discussed above and likely not the empirical ones, we and our colleagues recommend moving beyond the paradigm in which “discoveries” are made by single studies and single (thresholded) p-values. In particular, we suggest the p-value be treated, continuously, as just one among many pieces of evidence such as prior and related evidence, plausibility of mechanism, study design and data quality, real world costs and benefits, novelty of finding, and other factors that vary by research domain. In doing so, we offer concrete recommendations–for editors and reviewers as well as for authors–for how this can be achieved in practice; see McShane, Gal. et al. (2017).
Looking forward, we think more work is needed in designing experiments and taking measurements that are more precise and more closely tied to theory/constructs, doing within-person comparisons as much as possible, and using models that harness prior information, that feature varying treatment effects, and that are multilevel or meta-analytic in nature (Gelman, 2015, 2017; McShane and Bockenholt, 2017a, b), and–of course–tying this to realism in experimental conditions.
References
Benjamin, D. J., et al. (2017). Redefine statistical significance. Nature Human Behaviour. doi:10.1038/s41562-017-0189-z
Bartels, M. (2017). ‘Power poses’ don’t really make you more powerful, nine more studies confirm. Newsweek, 13 Sep. https://www.newsweek.com/
more-powerful-studies-664261Button, K. S., Ioannidis, J. P. A., Mokrysz, C., Nosek, B., Flint, J., Robinson, E. S. J., and Munafo, M. R. (2013). Power failure: Why small sample size undermines the reliability of neuroscience. Nature Reviews Neuroscience 14, 1-12.
Engber, D. (2016). Everything Is crumbling. Slate, 6 Mar. https://www.slate.com/
Gelman, A. (2015). The connection between varying treatment effects and the crisis of unreplicable research: A Bayesian perspective. Journal of Management 41, 632–643.
Gelman, A. (2017). The failure of null hypothesis significance testing when studying incremental changes, and what to do about it. Personality and Social Psychology Bulletin.
Gelman, A., and Carlin, J. (2014). Beyond power calculations: Assessing Type S (sign) and Type M (magnitude) errors. Perspectives on Psychological Science 9, 641-651.
McShane, B. B., and Bockenholt, U. (2017a). Single paper meta-analysis: Benefits for study summary, theory-testing, and replicability. Journal of Consumer Research 43, 1048–1063.
McShane, B. B., and Bockenholt, U. (2017b). Multilevel multivariate meta-analysis with application to choice overload. Psychometrika.
McShane, B. B., Gal, D., Gelman, A., Robert, C., and Tackett, J. L. (2017). Abandon statistical significance. https://www.stat.columbia.edu/
Open Science Collaboration (2015). Estimating the reproducibility of psychological science. Science 349, 943.
Simmons, J., Nelson, L., and Simonsohn, U. (2011). False-positive psychology: Undisclosed flexibility in data collection and analysis allow presenting anything as significant. Psychological Science 22, 1359-1366.
[/expand]
[expand title=”Commentary by Kiley Hamlin:”]
Should we lower the p-value that is considered statistically significant from the current p<.05 to p<.005? Benjamin et al. (2017) say we should, and estimate that doing so would require a 70% increase in sample sizes if we wish to maintain 80% power to detect significant effects. They note that p-values between .005 and .05 should still be published, but should be called “suggestive” rather than “significant”.
Many of the arguments raised in their paper are sound. Psychology (along with other social and natural sciences) does have an unacceptably low replication rate, probably due to researcher degrees of freedom that have allowed us to “p-hack” our way to p<.05 (Simmons et al., 2011). Clearly, this is a problem, and something needs to be done about it. That said, there are various reasons that I don’t agree that lowering the significance bar to p<.005 is the solution.
First, from my perspective we already have a solution. Eliminate researcher degrees of freedom, and get our false positive rate back to 5%. This can be done by spreading awareness of the dangers of post-hoc decision making, and having reviewers and editors ensure that all experimental and statistical decisions in accepted papers were made ahead of time. Clearly, the easiest way to accomplish this is preregistration, but submission checklists that include decision-making disclosures as well as highly vigilant reviewers/editors would also help a lot, inasmuch as they would ensure that everyone who isn’t a liar has stuck to the rules. Indeed, there are even methods that allow us to engage in (minimal) researcher degrees of freedom and then adjust for it, including sequential analyses (Pockock, 1977) and the use of p-augmented (Sagarin et al., 2014).
But, Benjamin et al. (2017) claim p<.05 is only weak evidence of a hypothesis anyways, whereas p<.005 is strong evidence, and so we should prefer p<.005 in the absence of p-hacking. Of course, everyone prefers their p-values to be .004 rather than .04, but it seems to me that one study is always going to be one study irrespective of its p-value. Perhaps a more fruitful change would be to require a direct replication, which also shows evidence at the p<.05 level, before one could abandon the phrase “suggestive evidence” in a paper. Indeed, although this essentially requires doubling one’s sample size, and so is in fact a bigger burden than the 70% average increase required by the p<.005 rule, one would be collecting more subjects with some evidence that doing so will pay off. My impression is that researchers would be more likely to get on board with this requirement than with p<.005.
These issues aside, by far my main concern with the proposal is that sample size increases influence various areas of psychology differently, and therefore the costs to researchers of this change will not be distributed equally. This is not a trivial concern or a whine about taking a bit more time to publish a paper: it would be unfortunate if researchers whose research questions lend themselves to the use of mTurkers or undergraduates were easily able to meet these new standards whereas the rest of us were not. It would also be unfortunate if researchers stopped testing hypotheses that are unlikely but potentially high-impact given the 70% bigger cost of doing so, and particularly unfortunate if our science were to get even WEIRDer (Henrich et al., 2010) than it already is because who has the resources to do “quality” science is increasingly clustered amongst a few well-funded researchers in highly populated liberal cities in the West. Indeed, it seems to me that this change would influence not only what we find out, but also who we find out about. Psychology needs to get better at these issues, not worse.
In contrast, given that p-hacking helps everyone find statistical significance, the costs of eliminating researcher degrees of freedom are far less likely to differ across fields, research questions, and researchers (at least costs will not differ more than what is already the case). In sum, I believe that eliminating p-hacking, perhaps combined with increased use of pre-publication direct replication, will be a more fruitful and less damaging way to improve psychology than reducing the p-value required for statistical significance.
References:
Henrich, J., Heine, S.J., & Norenzayan, A. (2010). The weirdest people in the world? Behavioral and Brain Sciences, 33(2/3), 1-75.
Pocock, S.J. (1977). Group sequential methods in the design and analysis of clinical trials. Biometrika, 64, 191-199.
Sagarin, B.J., Ambler, J.K., & Lee, E.M. (2014). An ethical approach to peeking at data. Perspectives on Psychological Science, 9(3), 293-304.
Simmons, J.P., Nelson, L.D., & Simonsohn, U. (2011). False-positive psychology: Undisclosed flexibility in data collection and analysis allows presenting anything as significant. Psychological Science, 22(11), 1359-1366.[/expand]
[expand title=”Commentary by Edouard Machery:”]
In Benjamin et al. (2017), we proposed to decrease the conventional statistical significance level from current .05 to .005. The main argument was that findings significant at the .05 level provided too little evidence for the rejection of the null hypothesis, and that this was one of the (not necessarily the, a fortiori not the only) factors underlying the current replication crisis in the behavioral sciences, epidemiology, and elsewhere.
The argument was put in Bayesian terms, but could have put in frequentist terms: A significance level at .05 would result in unacceptably high long-term frequency of false positives in the literature given the bias against the null.
To my surprise, our article has met with considerable resistance. While many objections and criticisms are well intentioned, they are de facto perpetuating the status quo. One of the virtues of our proposal is its practical simplicity: Its implementation only requires leading journal editors to agree on the new threshold. What we have to offer then is a simple fix that could improve the behavioral sciences quickly and substantially. Rejecting it on this or that ground without offering a similarly simple remedy to the woes that plague some fields in contemporary science is a recipe for perpetuating their crisis and the growing public distrust of science.
In what follows, I want to respond briefly to some objections that have been made in response to our proposal.
- The replication crisis is not merely, perhaps even not principally due to the .05 threshold
It is clear that many factors contribute to the replication crisis, and it is clear that decreasing the significance level threshold won’t address all of them. Benjamin et al. (2017) does not claim otherwise. But this seems to be a poor reason not to make the simple change we recommend.
- The proposal is easily applicable to fields that sample from on-line participants (e.g., Amazon Turk), but would be very difficult to implement in fields where it is challenging to obtain large samples of participants: developmental psychology, cognitive anthropology, neuropsychology, etc.
First, the proposal is not to require a p value lower than .005 for publication. If our proposal were accepted, journals could still publish results with a p-value larger than .005 (indeed larger than .05). Perhaps the only thing journal editors should take into consideration is whether the experimental and data analytic work is compelling, the hypotheses tested meaningful, and the conclusions drawn sound. So, developmental psychologists, cognitive anthropologists working in small-scale societies, and neuropsychologists would still be able to publish their work if our proposal were widely endorsed.
Second, the fact that it would be difficult for developmental psychologists, cognitive anthropologists working in small-scale societies, neuropsychologists, and others to meet a .005 significance level can’t be an argument against the claim that findings at a .05 level provide too little evidence against the null (or, in a more frequentist spirit, that the .05 level results in too many published false positives); it just shows that in some fields scientists are only able to collect a modest amount of evidence for their conclusions. This modest amount of evidence may be sufficient to warrant publication given the interest of the conclusions and the difficulty of obtaining more evidence, but that fact does not turn the modest amount of evidence they are able to collect into substantial evidence. Said differently, developmental psychologists, cognitive anthropologists working in small-scale societies, neuropsychologists, and others should not pretend to have stronger evidence than they really have. If they can only collect weak evidence, they should honestly say so.
- The proposal mistakenly proposes a single, conventional significance level; rather, the significance level should be set as a function of the costs of false positives and false negatives, which may vary from study to study or perhaps from field to field.
First, it would be a mistake to let scientists decide by themselves what the significance level should be in function of their perception of costs. This would add yet another degree of freedom in data-analytic procedures, which already suffer from too many degrees of freedom or forking paths. A conventional level alleviates this concern.
Second, in many areas of science, including much of psychology and neuroscience, the costs are nebulous and hard-to-specify, and it is hard to see how they could be taken into account.
- The proposal mistakenly proposes a significance level; rather, science should do without significance levels (just report the p-values or the Bayes factor).
Science is a social procedure that involves mechanisms by which phenomena are accepted. This is true in particle physics (5 sigmas significance level), epidemiology (consensus conferences and reports), climate science (Intergovernmental Panel on Climate Change), psychiatry (development of the DSM), etc. A consensual, conventional significance level is one of these mechanisms.
Reference
Benjamin, D. J., Berger, J. O., Johannesson, M., Nosek, B. A., Wagenmakers, E.–J., Berk, R., Bollen, K. A., Brembs, B., Brown, L., Camerer, C., Cesarini, D., Chambers, C. D., Clyde, M., Cook, T. D., De Boeck, P., Dienes, Z., Dreber, A., Easwaran, K., Efferson, C., Fehr, E., Fidler, F., Field, A. P., Forster, M., George, E. I., Gonzalez, R., Goodman, S., Green, E., Green, D. P., Greenwald, A., Hadfield, J. D., Hedges, L. V., Held, L., Ho, T.–H., Hoijtink, H., Jones, J. H., Hruschka, D. J., Imai, K., Imbens, G., Ioannidis, J. P. A., Jeon, M., Kirchler, M., Laibson, D., List, J., Little, R., Lupia, A., Machery, E., Maxwell, S. E., McCarthy, M., Moore, D., Morgan, S. L., Munafó, M., Nakagawa, S., Nyhan, B., Parker, T. H., Pericchi, L., Perugini, M., Rouder, J., Rousseau, J., Savalei, V., Schönbrodt, F. D., Sellke, T., Sinclair, B., Tingley, D., Van Zandt, T., Vazire, S., Watts, D. J., Winship, C., Wolpert, R. L., Xie, Y., Young, C., Zinman, J., & Johnson, V. E. (2017). Redefine statistical significance. Nature Human Behavior.
[/expand]
[expand title=”Commentary by Deborah Mayo:”]
1. The recommendation is based on an extremely old and flawed argument that’s been around since about 1960:
If you take the p-value–which is an error probability–and evaluate it by comparing it to a Bayesian measure which depends on a prior probability (and ignores error probabilities), then the two numbers can differ. The argument essentially turns on a well known fallacy:
That is, the p-value
Pr(test rejects H0|H0) can be .025 (or other number)
while
Pr(H0|H0 is rejected) >.025
if, for instance, it is assumed at least 50% of the null hypotheses are true–which is what they do.
This is called the fallacy of transposing the conditional. So they are comparing apples and motorcycles, and blaming the significance level for not being equal to a measure of something entirely different. Their argument either turns on commiting the fallacy or holding a Bayesian measure as a gold standard from which to judge a frequentist error probability..
Also over 60 years old are demonstrations that with reasonable tests and reasonable prior probabilities, the disparity vanishes. That is, the p-value matches or is close to the posterior. However, they still mean different things, and the Bayesian construal is far too strong..
2. The real trouble is, even if we grant them their priors–picked from we know not where– it turns out that the measure they recommend, the positive predictive value (PPV), or posterior probability of H1, actually greatly exaggerates the evidence! With high probability, they give high posterior to an alternative H1, even when H1 is false.
3. Now there’s nothing wrong with demanding a smaller p-value–provided you increase the sample size so that you don’t lose power. We should not be working with strict cut-offs for “significance” to begin with. Yet this paper encourages the idea of strict cut-offs.
4. Worst of all, this Bayesian move, at least on the systems of those I know in the list, ignores or downplays what almost everyone knows is the real cause of non-reproducibility: cherry-picking, p-hacking, hunting for significance, selective reporting, multiple testing and other biasing selection effects. Their Bayesian systems do not pick up on biasing selection effects or optional stopping (trying and trying again until you get significance). Thus, lowering the p-value while using their systems will do nothing to help, and will in fact worsen, the problem with false findings.
5. Finally, all this takes attention away from the real causes of non-reproducibility: the scientific inferences are not warranted by the statistical results. Every grade-schooler knows that statistical significance is not substantive significance, and yet their entire criticism is based on using significance tests as if they allowed this!
I discuss all of this at great length in my forthcoming book: “Statistical Inference as Severe Testing: How to Get Beyond the Statistics Wars” (Mayo 2018, CUP)[/expand]
[expand title=”Commentary by Neuroskeptic:”]
In their call to ‘Redefine Statistical Significance’, Benjamin et al. convincingly outline the weakness of the p<0.05 criterion.
They point out that under most reasonable assumptions, p=0.05 simply doesn’t correspond to very much evidence for the alternative hypothesis (H1):
A two-sided P-value of 0.05 corresponds to Bayes factors in favor of H1 that range from about 2.5 to 3.4… Conventional Bayes factor categorizations characterize this range as “weak” or “very weak.”
A p<0.005 threshold, Benjamin et al. say, does represent a strong evidence, and adopting it would only mean increasing the sample sizes required (to maintain 80% power) by about 70%.
Yet an objection could be raised against this change on the grounds of conservativism. If p<0.005 is better as a threshold, why then do all we use p<0.05? Surely, 0.05 was chosen for a reason. And surely the rules of probability haven’t changed since it was chosen.
If p<0.05 was good enough for Fisher, our statistical conservative might say, it’s good enough for me.
Benjamin et al. do sketch out a reply to this argument, saying that something has changed since Fisher’s time:
A much larger pool of scientists are now asking a much larger number of questions, possibly with much lower prior odds of success.
I think this point deserves more attention. Fisher introduced the p<0.05 significance threshold in 1925. This was a time when statistics was an activity carried out by hand. The electronic calculator, let alone the desktop computer, had yet to arrive on the scene.
After the manual calculation of means, variances, and statistics had been completed, the p-value corresponding to a given test statistic was generally looked-up using a printed table. So crucial were these tables that, in the 1934 edition of his 1925 book, Fisher included the following note:
With respect to the folding copies of tables bound with the book, it may be mentioned that many laboratory workers… have found it convenient to mount these on the faces of triangular or square prisms, which may be kept near at hand for immediate reference.
Clearly, our situation in 2017 is very different from that of Fisher in 1925. Instead of being artisanal works of labour, p-values are today mass produced, on demand. We have software that can carry out hundreds of statistical tests at the push of a button.
Whereas in Fisher’s day, a data analyst had to think carefully about which analyses were worth the time to carry out, it is now possible to carry out every comparison of potential interest and then, having seen the p-values, think about how to justify them.
In other words, there has been a quantitative change in the ease of calculating p-values, which has (I suspect) led to a qualitative change in the kinds of p-values we calculate.
Perhaps then, if we wish to continue using Fisher’s p<0.05 threshold in the way it was intended, we should consider dusting off our statistical tables and uninstalling our statistical software?
Neuroskeptic is a British neuroscientist who blogs for Discover Magazine.[/expand]
[expand title=”Commentary by Michael Strevens:”]
The assumption ostensibly driving Benjamin et al.’s proposal is that null hypothesis significance testing (NHST) presents an argument for the rejection of the null hypothesis. A high p value such as 0.05, they suggest, makes a weak case for “discovery”; only a lower value such as 0.005 ought to be regarded as making a strong argument that a new effect has been discovered.
There are numerous well-known problems with the notion that NHST provides an argument for hypothesis rejection or acceptance. Certainly, it does not provide a self-contained argument. At the least, it needs to be supplemented with, as Benjamin et al. recognize, “the prior odds that the null hypothesis is true, the number of hypotheses tested, the study design, … and other factors that vary by research topic”.
So why pretend that even a loose, prima facie distinction can be made between evidence sufficient for “discovery” and something that is “suggestive” but less than a discovery? Presumably, because if not presenting evidence for or against a hypothesis, what work could NHST be doing?
This rhetorical question, housing the assumption identified above, displays what I believe is a misunderstanding of the true role of NHST in scientific methodology. (By the “true role”, I mean the thing NHST does that makes science work, not the role attributed to it by statistics textbooks or scientific practitioners.)
The true role of NHST and many other statistical methods is to present putative evidence in a relatively neutral form and to note the likelihood (in the technical sense) of various hypotheses of interest on that evidence, given various auxiliary assumptions. Such a portfolio of evidence and likelihoods falls well short of an argument. But it performs a crucial function: scientists use the data and the likelihoods in the portfolio to make up their own minds as to what to believe, given their best prior estimates of the chance that various hypotheses, such as the null, are true, along with the broader empirical context. Different scientists bring different assumptions to the task, and so frequently evaluate the evidence differently, but the portfolio is nevertheless valuable to each scientist and therefore to the progress of science as a whole.
To interpret what is published in a journal as constituting an argument for null hypothesis rejection, for making a “discovery”, is to attribute to NHST something it cannot do. The same goes for using NHST to draw a contrast between evidence that is “suggestive” and evidence sufficient for a “discovery”. The only thing that matters, if I am right about the role of NHST, is the threshold for publication, since that is the difference between making the portfolio widely available and not doing so. (I don’t rule out the possibility that the threshold should be lower in some fields; the stringent standards in genomics and high-energy physics have certainly been salutary.) But the authors are not proposing to change the publication threshold. So I must conclude that their proposal will offer little benefit to the smooth functioning of scientific inquiry.
[/expand]
[expand title=”Commentary by Kevin Zollman:”]
One of the central features of social norms – both inside and outside of science – is to counteract selfish motivations. Scientists are driven by many considerations. While the search for truth is among them, researchers are also subject to self-serving biases and have career aspirations. So motivated, a scientist might try to publish something which, we as a society think they ought not. To deal with this conflict between individual and collective interest, we invent scientific social norms like the 0.05 threshold for p-values.
One might think scientists ought not be so motivated. Scientists shouldn’t care for their careers, one might say. Even if this were possible, I would not advocate for it. The selfish motivations of scientists have important positive consequences of their own, and on balance science might do worse by changing them. We might want to change the relationship between publication and career rewards, but such changes would be far more revolutionary than what we are discussing now.
If we are not changing scientists’ motivation to publish, we must ask if our current social norms effectively align individual and collective interests. The authors of the target article think not. Scientists are asserting claims which we prefer they not. To combat this, we should reduce the threshold p-value for publication.
Changing social norms can be a tricky matter. An apparently reasonable change to the Caribbean Cup Soccer Tournament rules in 1994 created a system with remarkably counter-intuitive consequences. As time ran out on the Barbados-Grenada match, Grenada discovered that it served their interest to score a goal in either net, including their own. For the last seven minutes, Barbados was forced to defend both goals while Grenada tried to kick a goal in either one. Needless to say, this was far from the intentions of tournament designers.
Is there an analogous consequence in the scientific case? To answer this question, we must investigate those problems the authors hoped to set aside. Scientists find many ingenious ways to publish what would ordinarily be unpublishable. They shop around for favorable statistical tests or check many variables simultaneously looking for a favorable result (or one of a litany of other cunning strategies).
The authors correctly note that the change in the threshold p-value does not “substitute for” solutions to these other tactics. While true, this is far too weak a statement. In the absence of appropriate countermeasures, changing the p-value may very well encourage more manipulation of statistical evidence. Scientists would likely increase their use of these other nefarious strategies in order to publish.
How bad would this be? After all, reducing the threshold p-value removes one avenue for publication. So, it must improve things, right? I think this is also too quick. As Dan Malinsky points out, scientists will change what data they collect and how they analyze it. This may introduce new or worse errors than before. And, under the authors’ proposal, these errors bring with them a more convincing p-value. This affords the results greater trust from the scientific community and public at large. All this might leave us in worse epistemic situation than before. Or it might not. It’s genuinely hard to say.
In the end, I am not advocating that we should keep the current threshold p-value. Nor am I saying that we should change. I am concerned that without a more systematic study of the interacting incentives, we just might encourage the scientific equivalent of an own-goal.[/expand]
It is also very important to remember that Fisher did not see p < .05 as a sufficient condition for truth, reliability, or publishability. See the Fisher quote in https://www.psychologicalscience.org/observer/taking-responsibility-for-our-fields-reputation
“No isolated experiment, however significant in itself, can suffice for the experimental demonstration of any natural phenomenon” (Fisher 1937, p. 16). Quote taken from our literature review on significance thresholds: https://peerj.com/articles/3544
Together with Sander Greenland, I suggest to “Remove, rather than redefine, statistical significance” https://rdcu.be/wbtc
I agree with the statement that no isolated experiment is sufficiently demonstrative, but I would argue that no experiment (no matter how novel) is conducted in isolation. That is why we need Bayesian priors. In my blog, I argue that every study should be analyzed as if it were part of a single study meta-analysis.
In most cases an empirical studies conducted in the social sciences constitutes a single-study meta-analysis such that we lack the data to estimate the moments of a study level distribution of the treatment effects. We are therefore compelled to make assumptions about location, variance, and even the form of this distribution. In most cases, the choice for the study level variance of the effect size should be based entirely on subjective evaluations of the prospecttive evidentiary value (i.e. external and internal validity) of the study. Choosing a small variance for the prior assigns a greater proportion of the total study variance to within-study variance – yielding more within study error (design error + sampling error) and more shrinkage – usually towards a skeptical or null conclusion. The advantage of the Bayesian approach over a version of the NHST framework which prospectively chooses a lower Type 1 error rate for studies that suffer from design flaws – is that one can (somewhat principally) talk about “levels” of significance using the full posterior distribution rather then simply drawing a binary significant or not significant conclusion. I am agnostic about the accuracy of these more nuanced conclusions, but researchers are going to make them anyway so we might as well make the process transparent!
Fisher said almost 100 years ago, in 1926
“Personally, the writer prefers to set a low standard of significance at the 5 percent point. . . . A scientific fact should be regarded as experimentally established only if a properly designed experiment rarely fails to give this level of significance”
So it isn’t fair to blame him for the P < 0.05 myth.
That is a daft idea. Throw out p-values and insist on grownup Bayesian stats.
This. Every time.
I have also replied to Benjamin et al. See pp 18 – 19 of
https://www.biorxiv.org/content/early/2017/08/07/144337
I commented in a blog post from the perspective of Neyman Pearson’s distinction between type-I and type-II errors.
https://replicationindex.wordpress.com/2017/08/02/what-would-cohen-say-a-comment-on-p-005/
In my own blog, bigsciencesite.wordpress.com, I propose a Bayesian analog to the idea of lowering the Type 1 error rate. Please check it out!
I fear that a subjective Bayesian solution will never work. I agree with Valen Johnson. when he said
“Subjective Bayesian testing procedures have not been —and will likely never be — generally accepted by the scientific community [25]”
On the other hand. one certainly can’t ignore priors. I advocate Matthews’ solution. Calculate the prior that you’d have to believe in order the achieve a false positive risk of, say, 5%.
.
David, could you provide full citations for Johnson and Matthews. I don’t know if you read my blog post, but I advocate for a subjective Bayesian (or “fully” Bayesian) inferential framework in my blog. I would love your feedback! My proposal sounds similar to Matthews solution. One specifies the prior that is consistent with an effect size that is associated with a posterior density where the null hypothesis (location of a parameter) is implausible (< 5%). In other words, we ask in advance what effect size would you need to see from this PARTICULAR study in order to conclude that a zero effect (for example) is really unlikely. This assessment would be based on their own subjective assessment of the evidentiary value of the study. While the scientific community may not accept fully Bayesian inference, I think they must accept some way of formalizing their subjective evaluations of the evidentiary value of a study PRIOR to seeing the findings. The need to do this is at the heart of testing PREDICTIONS – and doesn't require Bayes theorem to justify.
The full citations for Matthews, Johnson etc are all in my paper!
https://www.biorxiv.org/content/biorxiv/early/2017/08/07/144337.full.pdf
I’d appreciate your opinions on my approach. It’s all a lot simpler if you use a point null. Some people don’t like them, but I argue (in appendix A1) that they are a natural thing to test.
I’ll look at your blog. I certainly agree that you can’t ignore priors, hence my suggestion -hence my suggestion that P values should be accompanied by a statement of the prior that you’d have to postulate in order to achieve a false positive risk of, say, 5%.
Thanks David! I will certainly read your paper!
I like your post in principle. But I just can’t believe in informative priors. They just corrupt analyses with your prejudices. That’s why I prefer (if x is true effect size) H0: x=0, H1: x |= 0
David, thanks very much for the feedback. I have not really found anybody who likes the idea very much. I have not had a chance to read your paper, but will do so soon!
The xkcd cartoon is wonderful, but every time I see it I find it remarkable that no-one asks what we would like the statistical results to look like if green jelly beans, and only green jelly beans, cause acne. The answer is exactly what they look like in the cartoon.
The ‘solution’ to most of the problems that people seem to have with P-values and statistical ‘crimes’ is to require corroboration of all important findings. Corroborative experiments are routinely done in some areas of science, but they are alarmingly absent in others (psychology, I’m looking at you!).
Replication is just an easy way to achieve transparency in confirmatory studies because it is an easy way to achieve consensus on study design (what outcomes to measure, how to manipulate the exposure, etc.) and significance to the field (e.g. relation to established theory or other empirical results). The original study may have figured all of this stuff out, but in the dark. All that is left is to come to a consensus about whether a new study constitutes a valid replication – prospectively of course. The problem in many fields is that there are severe constraints on replication. For example, once a single clinical study has established that a certain drug is more effective then another drug (or placebo), it is unethical to replicate this study in a human population. You get one shot. Corroborative experimentation is severely constrained.
It’s true that in many fields there are impediments to replication. However, we need to not confuse ‘replication’ with ‘corroboration’. Replication usually denotes a new study of the same phenomenon carried out by a third party. Replications are necessary on occasion. Corroboration should be contained within _every_ scientific study in which it is possible. Corroboration can easily be achieved in the jelly beans cartoon by simply re-testing the green jelly beans. (I note that the original presentation of the cartoon mentions a failed re-testing of the greens in a usually unnoticed mouse-over.) Given the size of the original study (21 units), a requirement to retest one unit is hardly onerous.
If direct corroboration is not possible then indirect corroboration from other observations or even from theory may be possible, and would be far better than a reliance on a single P-value. If corroboration is not possible then the study is phenomenology rather than science.
I’m so glad to see comments. It’s in the biomedical fields where exceptional energy will be forthcoming. With these world-wide consortias, I think there are collaborative synergies to be gained.
It is substentially a matter of discipline on top of the logic of validity and falsification. If Theory is important then the accurate level of P value, whether smaller than 0.05 or 0.001 etc. is less important. And then we should seriously consider the advantage of Bayesian statistics. Great and important debate but also should be conducted in the context of the relevant discipline
“When Theory is important…”. I interpret this as meaning in areas/discipline where there is wide spread consensus on the prospective evidentiary value of empirical studies (I.e. when everybody knows what is at stake because the study design is well connected to established theory.) When there is a lack of consensus or when the connection between study design and theory is weak, then we need a transparent way of incorporating varying levels of credulity (subjective) into the inferential framework. Bayesian inference is one possibility, but no the only one…